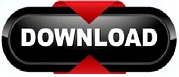
The variables are resp, the number of victims the respondent knows, and race, the race of the respondent (black or white). The data are from a survey of 1308 people in which they were asked how many homicide victims they know. The data are presented in Table 13.6 in section 13.4.3. To illustrate the negative binomial distribution, let’s work with some data from the book, Categorical Data Analysis, by Alan Agresti (2002). Say our count is random variable Y from a negative binomial distribution, then the variance of Y isĪs the dispersion parameter gets larger and larger, the variance converges to the same value as the mean, and the negative binomial turns into a Poisson distribution. The variance of a negative binomial distribution is a function of its mean and has an additional parameter, k, called the dispersion parameter. This suggests it might serve as a useful approximation for modeling counts with variability different from its mean. Unlike the Poisson distribution, the variance and the mean are not equivalent. The negative binomial distribution, like the Poisson distribution, describes the probabilities of the occurrence of whole numbers greater than or equal to 0. One approach that addresses this issue is Negative Binomial Regression. Performing Poisson regression on count data that exhibits this behavior results in a model that doesn’t fit well. When we see this happen with data that we assume (or hope) is Poisson distributed, we say we have under- or overdispersion, depending on if the variance is smaller or larger than the mean. A distribution of counts will usually have a variance that’s not equal to its mean. While convenient to remember, it’s not often realistic. However one potential drawback of Poisson regression is that it may not accurately describe the variability of the counts.Ī Poisson distribution is parameterized by \(\lambda\), which happens to be both its mean and variance. For example, we might model the number of documented concussions to NFL quarterbacks as a function of snaps played and the total years experience of his offensive line. This is a generalized linear model where a response is assumed to have a Poisson distribution conditional on a weighted sum of predictors. When it comes to modeling counts (ie, whole numbers greater than or equal to 0), we often start with Poisson regression.
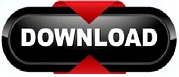